This article is the first in our article series titled “Wearable Devices for Lifestyle and Medical Monitoring”. Readers can access the other articles here:
Section 1: “General Problems in Existing Health-Care System and How Personicle Addresses These Gaps”
Section 2: “Details of Device Ecosystem, Type of Data Being Generated and Why These Architectural Decisions”
Section 3: “How Personicle Addresses Technical Challenges in Leveraging Data from Wearable Devices”
The Need for Incorporating User-Generated Data
Wearable devices are one of the main ways we can empower health care providers as the population worldwide heads toward more and more unhealthy lifestyle choices, especially in First World Countries. They offer solutions for remote patient monitoring and patient care (including physician-controlled drug administration) unlike anything we’ve seen in the recent past. According to AAMC (Association of American Medical Colleges), there is estimated to be a shortage of 37,800 – 124,000 physicians in both the primary care and specialty-care sectors by 2024(source). The solution to this would be using wearable devices to closely monitor and treat patients, saving time and increasing the effectiveness of healing modalities due to the shortage in physicians, exceeding healthcare costs, and an increase easily preventable chronic and lifestyle diseases both at home and in hospital. If implemented safely and with required health care protocols in mind, the outcome would be more patients being treated from home and there being more vacancies for hospital beds as well as round-the-clock monitoring of patients’ vitals from any location with higher efficiency rates, as well as early detection and diagnosis of diseases – both chronic and acute – personalized to individual patient physiology. Wearable technologies are becoming increasingly accurate and predictive, and combining them with machine learning algorithms can allow us to predict diseases that would otherwise remain asymptomatic and undetected before they could be detected or diagnosed clinically in the hospital; this would save patients money as they could seek cheaper and more effective treatment options in lieu of more expensive and lengthy treatments which would be the only alternative if they are diagnosed at a stage where their disease has progressed significantly. The feature of having more patients be treated from home would have impacted the healthcare industry enormously during the COVID-19 pandemic, allowing physicians to monitor patients from home while they recovered without having to overcrowd the hospitals. Perhaps one of the most insightful features of using wearable devices and the advantage it provides to doctors is data collected on individual physiological systems – including respiratory, skeletal, nervous, renal, immune, endocrine, cardiovascular, digestive, reproductive, and muscular systems.
The healthcare sector is looking to incorporate user-generated data, which will result in tremendous leaps both fiscally and as it pertains to human health. According to statistics from Gartner, wearable devices spending was $69 billion in 2020 and reached $81.5 billion in 2021, increasing end-user spending by a whopping 18.1%, and this trajectory is projected to continue. Noteworthy purchases include ear-worn devices which grew 17.6% to $21.8 billion in 2020.
The monetary impacts of using such methods on the current health care system are visible not just as earnings but also from a savings vantage point. According to the Centers for Disease Control and Prevention, 51.8 % of all Americans have one or more chronic conditions attributable to poor diet, lack of exercise, and stress as of 2018.2 These conditions are the leading drivers of annual health care costs in the U.S., approximating $3.8 trillion (or 84%) of health care spending (source). Data from wearable devices can be used to manage chronic conditions caused by lifestyle by instructing, guiding, and informing users’ day-to-day choices. U.S. employers insure 170 million people and spend $12,500 in premiums for employees and their families. These premiums are expected to rise to $24,500 by 2025 and the majority of it can be prevented by getting control over chronic lifestyle and preventable diseases, saving employers a substantial amount of money. Wearable devices can enhance clinical care and contribute to savings through reduction in obesity, food and exercise management, better monitoring of chronic health conditions, and fostering closer relationships between health-care providers and patients.
In the next 25 years, wearables are projected to save $200 billion on health care and lower hospital costs by 16% in 5 years’ time resulting in lowered operating costs for hospital CEOs. Insurance companies are also positively affected as fewer claims will be filed because of incorporating wearable tech into insurance plans due to healthier clients. Insurance companies can leverage the functionality of having personal records, reports, and bills sent directly through an application to the family, making the process of clearing insurance claims much easier, as well as processing costs and disbursal of money on a priority basis.
The monetary impact of such methods also extends to the manufacturing, sales and tech industry. The widespread market adoption of wearable devices has resulted in a rapidly growing consumer segment. A digital ecosystem of stakeholders including but not limited to end users, health care professionals or organizations, emergency services, and insurance companies has emerged. By working together, these stakeholders can derive substantial benefits from collecting data pooling, sharing, and cross-correlation resulting in new insights. Third-party companies can also get involved in motivating patients to perform specific tasks through the offering of incentives and gamifying achievements, resulting in revenue generation while molding user behavior. Discount coupons for health care items drive revenue by encouraging users to buy the items.
Wearable devices will also play a hand in saving a sizable portion of time. In the past, deficiencies in communication between medical hardware, counterpart software solutions, and medical EHR (Electronic Health Record) systems was a rate-limiting factor in the desire to phase out episodic health care and replace it with continuous patient care. By using wearable devices to collect substantial amounts of data, the health care sector can use present EHR systems to mount and escalate the health sector in terms of interoperability. This will result in successful and efficient communication, dissemination, sharing, and availability of knowledge among constituents, transforming the health care sector into an integrated system. Several therapeutic wearables such as Zephyr’s Anywhere BioPatch allows tracking patient vital signs and physiology minute-by-minute instead of in an eight-hour window which would be the alternative option without wearable technology.
2019 | 2020 | 2021 | 2022 | |
---|---|---|---|---|
Smartwatch | 18,501 | 21,758 | 25,827 | 31,337 |
Wristband | 5,101 | 4,987 | 4,906 | 4,477 |
Ear-worn | 14,583 | 32,724 | 39,220 | 44,160 |
Head-mounted display | 2,777 | 3,414 | 4,054 | 4,573 |
Smart clothing | 1,333 | 1,411 | 1,529 | 2,160 |
Smart patches | 3,900 | 4,690 | 5,963 | 7,150 |
Total | 46,194 | 68,985 | 81,499 | 93,858 |
Personicle and clinical health ecosystem
There are various gaps in the existing healthcare ecosystem that can be alleviated by leveraging user data generated from wearable devices and a system like Personicle, we will discuss some of those in the subsequent sections:
No View of Patients Between Visits
Personicle is addressing well-being and lifestyle challenges through providing healthy lifestyle choices and diet suggestions to patients as a complementary component to physician care. There are unfortunate downsides to patients meeting with doctors a few times annually and there being no patient monitoring in-between visits. A patient’s nutrition, activity level, sleep hours, stress, and lifestyle can change drastically from one day to the next and these factors can severely impact their vitality and health (either in a limiting fashion or in a healing fashion). According to 81.8% of studies as part of a meta-analysis, if doctors had intermittent or continuous views of a patient’s health status, it would cause statistically significant reductions in mortality all by increasing continuity of care source.
Personicle tackles these issues and surpasses them by leaps and bounds, offering 365 days a year of round-the-clock monitoring and creating pools of data lakes that can be parsed, manipulated, and mined to look for the necessary information that aids patients in improving their lives. Creating visual representations of habits through the passive collection of data and displaying important statistics (such as a user’s sleep trends or mobility score) is much more useful to a doctor than patients being asked questions and recollecting from memory. Self-reporting from memory has the added downside of patient’s tampering with data by lying, or accidentally misrepresenting information. This effectively resolves the issue of having no view of patients between visits. The continuous view of patients between visits is mut more beneficial than having only a snapshot, both for patients and doctors. Making passive data collection part of the decision-making process gives great insights.
Personicle can trigger Ecological Momentary Assessments (EMAs) to collect data in specific situations such as feedback about mood on cloudy days with low visibility, as well as information about exposure to environmental factors such as pollution. It allows physicians to give patients exercises and real-time recommendations, programmed directly into the device and complete with daily reminders, based on their current or future activities to improve their overall health and choice-making. This continuous view of the patient’s health provides better infrastructure to support health habits alongside prompts from EMAs.
Passive sensing as opposed to manual input of data by users is a feature desired by health care practitioners and requires addressing challenges on the user side about compliance, user fatigue, intelligent EMAs, and notifications. Intelligent EMAs require context recognition capabilities and can be triggered in situations to obtain detailed information about user activities. For example, if the user is eating in a restaurant Personicle can trigger an EMA to log your meal. EMAs will allow physicians and patients to log symptoms as soon as they experience them rather than having to recollect them down the line during a clinical visit. This is useful for the end-user because it reminds them that this is important information, and it will be beneficial to log it in the present moment. An example specific to clinical utility is EMAs triggered by the user logging certain events representing symptoms (such as migraines) into Personicle. Doctors can associate a subset of questionnaires with such symptoms. This will send a notification to the user asking for detailed related information (How long has it been since your last meal? Were you exposed to loud noises? Are you currently experiencing digestive issues such as IBS? Are you dehydrated?). Such a logging mechanism could provide more detailed and accurate information than the existing procedure of clinical interviews and questionnaires.
The aggregation of all this data allows physicians to go from a single snapshot of the person to a continued view of their symptoms and activities. A lot of the activities are derived from wearable devices but Intelligent EMAs can be used to record a user’s symptoms and disease progression. It must be stressed that the value that wearable device data gives is providing various benefits and insights to the end-user and patients that would not be the case without Personicle.
Antiquated methods for lifestyle data collection
Outdated methods for lifestyle and data collection include surveys, forms and user-reported lifestyle data which are all prone to errors. These methods are susceptible to many statistical and methodological errors including:
Coverage error: Unknown or zero chance of individuals in the population being included in the sample. Reducing the error involves implementing a multimodal design.
Sampling error: Sampled individuals not representing the characteristics of the population. Reducing the error involves clearly choosing and identifying a population of interest using diverse participant recruitment strategies and large, random sample sizes.
Measurement error: Questions or instruments that do not accurately reflect or measure the topic of interest by injecting bias. Reducing the error involves using reliable instruments, pretest questions, user-friendly graphics, and visual characters,
Nonresponse error: Lack of response from individuals in the sample. Reducing the error involves using only user-friendly survey designs and follow-up procedures for participants who have not responded (source).
Health Risk Assessments (HRAs) collect self-reported data from patients and give professionals insight into how to improve the health of the population. They are very complementary to biometric or clinical data gathered by primary health care providers. Minimizing bias and collecting quality, reliable self-reported data involves addressing social desirability bias, addressing recall issues, and avoiding assessment fatigue. Social desirability bias can be addressed by providing private environments and securing data under the law as well as disclosing how data will be used to ensure that participants don’t feel judged by others or disqualified from health coverage. Recall issues can be solved, and the participant can give a recount with 90% accuracy rate if the recall is within one month or less of the event. Finally, assessment fatigue in participants can be minimized by asking the smallest number of questions in simple words. Dynamic branching logic avoids assessment fatigue by only posing questions based on answers to previous questions.
These errors in lifestyle data collection put additional burdens on patients as well as providers. Patients are required to put in additional effort to recall their life events and fill in surveys at every doctor’s visit. Doctors also need to go through this information in a text format instead of easy-to-understand charts and visualizations. Continuous passive monitoring using sensors and wearable devices would resolve this issue.
Accountability and healthy habit promotion
In order to make sustainable and healthful lifestyle behavioral modifications, patients need to be held accountable for their choices. The current healthcare paradigm does not have an accountability mechanism. This in part can be attributed to the issue of having no information about patients between visits.
Sharing of Personicle data with providers and family members can help set up accountability mechanisms. People in your life who are aware of your habits or needs can use this information to understand what kind of habits need to be promoted for you to live a healthier life. No one understands your life more than family members, partners, friends, or work colleagues. People in your life are the best accountability mechanism you can have. Providing these individuals with supplementary Personicle data can assist both them and the patient in helping the patient acquire healthier habits. Group activities such as working out together, going hiking together, or becoming walking buddies are a great way to adopt healthier habits in a sustainable and enjoyable way.
The effectiveness of various interventions in changing specific health behaviors has been studied, both in terms of promoting health-promoting behaviors and curtailing bad habits. A wide body of evidence has illustrated that many interventions have effectively achieved behavior change across a range of health behaviors. The most successful interventions include workplace interventions to support smoking cessation, physical activity, and healthy eating; school-based interventions across the health behaviors; individual-level interventions drawing on physician advice to promote healthy eating, smoking cessation, and responsible/safe levels of alcohol use; and counseling for tobacco and alcohol use (source).
Medication usage and adherence are irregular and unreliable, with a nearly 50% non-adherence rate of patients not taking their prescribed medications. However, the sole responsibility for this does not fall on the patient. Physicians, patients, and process components must work together to regulate patient adherence to taking medication, a complex process to implement. Strategies that can be used to overcome barriers to medication adherence can be broken down into patient-related, physician-related, and health-system related factors. This includes health literacy, addressing mental health issues, invoking patient participation, addressing financial issues and access to care, Medicare plans, National Council on Aging, awareness, communication, dosing complexity, time constraints, lack of care coordination, and lack of automation(source).
According to a series of 10 studies with 12,414 participants, interventions resulted in an increase in fruit, vegetable, and fiber consumption and decreases in exogenous fat consumptionsource. Several randomized trials have evaluated the potential to modify patients’ dietary habits through primary-care based interventions.
Personicle can help promote healthy habits among the users including medication adherence and dietary choices. The collected data can be used to identify a model of the user behavior and understand their actions in distinct circumstances.
The “Fogg Behavior Model” can help us better understand how patients can be motivated by Personicle to make health-producing decisions when prompted. To get change in behaviors to occur, the 3-element triad of motivation, ability, and a prompt must converge at the same moment. If the behavior does not take place, then 1 of the 3 elements is missing.
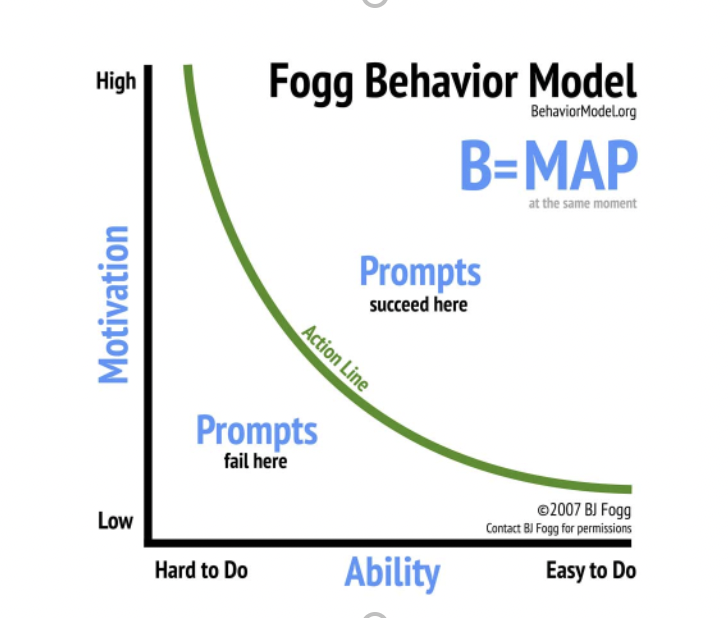
The issue encountered and seen with the Fogg Behavior Model is that someone can be prompted (reminded) to do something as well as have the desire (motivation) to follow through, but not the power (ability) to do so during the present moment. The solution to this is to create an integrated system so that Personicle can synchronize with calendars, Google maps or GPS, wearable devices, alarm clocks, and more to offer real-time solutions to the user when they are prompted to make a decision. This enables providers to promote healthy habits as the Fogg Behavior Model can be used to identify proper triggers based on individual motivations.
Conclusion
The episodic clinical care paradigm, while effective against infectious diseases, has proven inadequate for managing chronic illnesses. This is largely due to the lack of a continuous view of patients’ lifestyles and habits (as these diseases are mainly driven by lifestyle choices), and overt reliance on population-level controlled and randomized clinical trials for clinical decision making. The knowledge and insights derived from such studies are valuable for understanding diseases and biological phenomena but fail to capture individual treatment variability (source). We can overcome this limitation by adopting evidence-based medicine at an individual level that treats every patient as a unique person. Personicle is an essential step toward a data-driven person-centric healthcare paradigm that presents a holistic view of a patient’s life and brings the focus on the patient rather than the disease.